Machine Learning: A.I. That Works
A.I. has been around for a long long time, but where does it stand now? Is it dangerous? What exactly is it and why do we need it?
A Bit of History on A.I.
The concept of Artificial Intelligence is found in stories and literature since ancient times. It had become more and more present starting with 19th century Sci-Fi literature, even before scientific ways of trying to achieve it were discovered.
A.I. is an evolving concept, whose definition is constantly being updated. A.I. is something artificial that is capable of mimicking or reproducing human thought processes or even consciousness. The definition is being updated as computations for example, who were considered A.I., are now very common and therefore are not considered A.I. anymore. Same for a device that emulates eyesight for example, which being common and done for the past decades, it was removed from the concept of A.I.
General A.I. and the Fear of Machines Taking Over
The broader definition of A.I. is the so-called General A.I., an A.I. that would be capable of doing most human functions, or even more. The correct name is Artificial General Intelligence (A.G.I.), but is more commonly referred to as General A.I.
This is the broader type of A.I. usually portrayed in Sci-Fi. Books like Isaac Asimov’s ‘Robot’ series, TV series like ‘Star Trek: The Next Generation’ and ‘Westworld’, movies like ‘2001 A Space Odyssey’ and the more recent ‘Her’ and ‘Ex Machina’ or even video games like the ‘Mass Effect’ series or ‘Destiny’ trilogy, have made A.I. part of scientific pop culture. This is the reason why this is the very type of A.I. most people associate A.I. with.
Some of these fictional universes even feature entire alien races of robots controlled by A.I., like the Borg in the Star Trek universe, the Cylon in Battlestar Galactica, the Geth and Reapers in Mass Effect or the Vex in the Destiny universe. These races of robots are usually portrayed as enemies and they oppose our very humanity in long epic wars.
In the ‘Terminator’ series, A.I. developed by humans turns against humanity and a war wages. The war is eventually lost by humans. A.I. has been regarded as a threat to humanity since inception, but that does not have to be the case.
Machine Learning, a Practical Way of Building an A.I.
‘Machine Learning’, abbreviated M.L., is a branch of computer science that tries to program machines that can learn by themselves after being initially programmed. ‘Machine Learning’ is a newer concept, but not as new as you might think. You may have just heard about M.L. because of the fantastic progress that was done in this field in recent years, but the term was coined at the end of the 50s by Arthur Samuel, a pioneer of computer science working at IBM.
So, how does it work?
Machine Learning is based mostly on statistics and probabilities. You construct a model and define some parameters for that model. Based on those parameters, the machine will make predictions with a certain degree of probability. You will then feed different sets of data to the model, to refine the decision making and make the model make better predictions. This is called training. After enough sets of data are fed into the model and the model refined, the machine will be able to adjust to new sets of unseen data by itself, using the model created and refining it as more data is collected.
The more sets of different data initially fed into the model, the more accurate the model will be. You can imagine why training such models by hand was very difficult in the 1950s. Progress was slow because of the lack of sufficient data and because of the lack of computational power for the respective data.
The growth of computing power after the 2000’s, the rise of the Internet and therefore the collection of Big Data and the money invested by rich companies like Google or Tesla, interested in such research, are the key factors that lead to the recent major leaps in the field.
Machine Learning, a Better Marketing Brand
Is Machine Learning something different from A.I.? Well, it is, and it is not. You may regard Machine Learning as a type of A.I., given there are other methods of trying to make an A.I., like Symbolic Artificial Intelligence. But at the same time, M.L. is sufficient by itself to build a fully standalone A.I. However, the term itself is less important that what it tries to achieve.
Because the term A.I. is associated to a fully capable General A.I. in the collective mind, as a marketing decision ‘Machine Learning’ is more specific and less scary of a term. Therefore, marketers have abandoned the term of A.I. for their products and prefer Machine Learning or Deep Learning instead, as it is more specific and creates less of a confusion or a paranoia in the minds of customers, the press, or potential investors.
Neural Networks and Deep Learning
Neural Networks (N.N.) are networks of data that try to emulate the human brain. They are organized in nodes, each node acting as a neuron acts in the human brain. The relationships between nodes are defined using advanced Calculus.
As computers evolve, larger neural networks are being created and stored. The more layers in a neural network, the deeper that neural network is. Using Machine Learning on deep Neural Networks, and adding layer and layer to that same network, you get more accurate Machine Learning models. This is colloquially referred to as Deep Learning, although different researchers use different names for their Neural Networks and methods, some even choosing different names simply for marketing reasons.
Many of these models are made public, some collected in huge libraries that can be accessed, while others are not made public. Different researchers use different training techniques for their models, or different constructions of Neural Networks that better suit their use case.
Deep learning is in its pioneering phase, and roads are less traveled than those of other fields of computer science, many researchers therefore rooting for a model to other researchers or to the public.
Andrew Ng, Chief Scientist at Baidu, posted this graph in a slide that shows why Deep Learning is better than older learning algorithms. Also check out his website andrewng.org.
A.I.: Can It Make Me Better Coffee?
My answer to this question is a plain and simple yes. Machine Learning can improve crops of coffee plants by learning their growth conditions and then adjusting optimal light and water to the plant to make better beans, and make you coffee from those beans.
Then, using a video camera, the coffee maker scans your expression and knows if you liked that coffee more than another coffee it made, and in time it can slowly make you the best custom coffee. Also, it scans your blood pressure and makes you coffee that is stronger based on your current blood pressure. It adjusts taste parameters accordingly, so that no matter the blood pressure and therefore the amount of caffeine in your cup, the taste will be like any other day when the blood pressure was different. And if your coffee maker is connected to the internet, it will also upload your medical data that it recorded to your medical file in the cloud.
This was a use case for a simple process like making coffee. You can imagine more ways of improving your coffee using machine learning, feel free.
I recommend this Tedx by Maurice Conti that shows more use cases, some already developed, some under development. It paints a vision of the future alongside A.I., a man-machine symbiosis which he calls the augmented age. It is a more likely and more pleasurable scenario than being annihilated by rogue A.I.s.
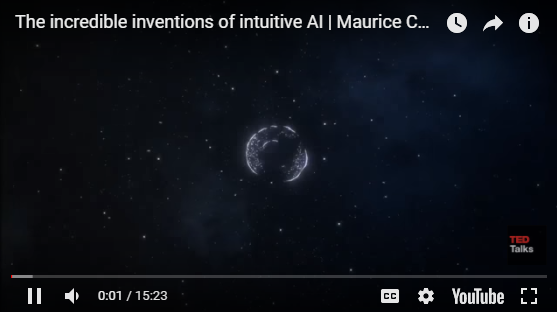
So, How Long Before a Robot Falls in Love with My Wife?
We discussed several months ago about A.I. taking your job.
I am just as skeptical as last time about the development of Strong A.I. and most experts that are actively involved in the development of Neural Networks share my opinion.
However, given the complexity of the issue at hand, no one really knows when the synergies between different Neural Networks connected to each other, alongside some other future breakthroughs, could lead to the birth of a General A.I.
I don’t believe this will be anytime soon, but caution is advised, and research done by institutes like M.I.R.I. (Machine Intelligence Research Institute) are most welcome. Eliezer Yudkovsky is one of the founders of M.I.R.I. and his research touches various subjects, including how to design moral A.I. I suggest following Eliezer on Facebook or Twitter.
Aside from technical information, his philosophical views are just as interesting, as Isaac Asimov’s ‘Three Laws of Robotics’ are not sufficient at this stage of A.I. development, and we require a more scientific approach.
I do not know when a robot will fall in love with your wife, therefore I recommend constant monitoring of the subject, using implanted chips. Kidding, better not be jealous or some machine at some point may learn that horrible emotion from you.
Author: Tiberiu Jakab